Kyle Lo
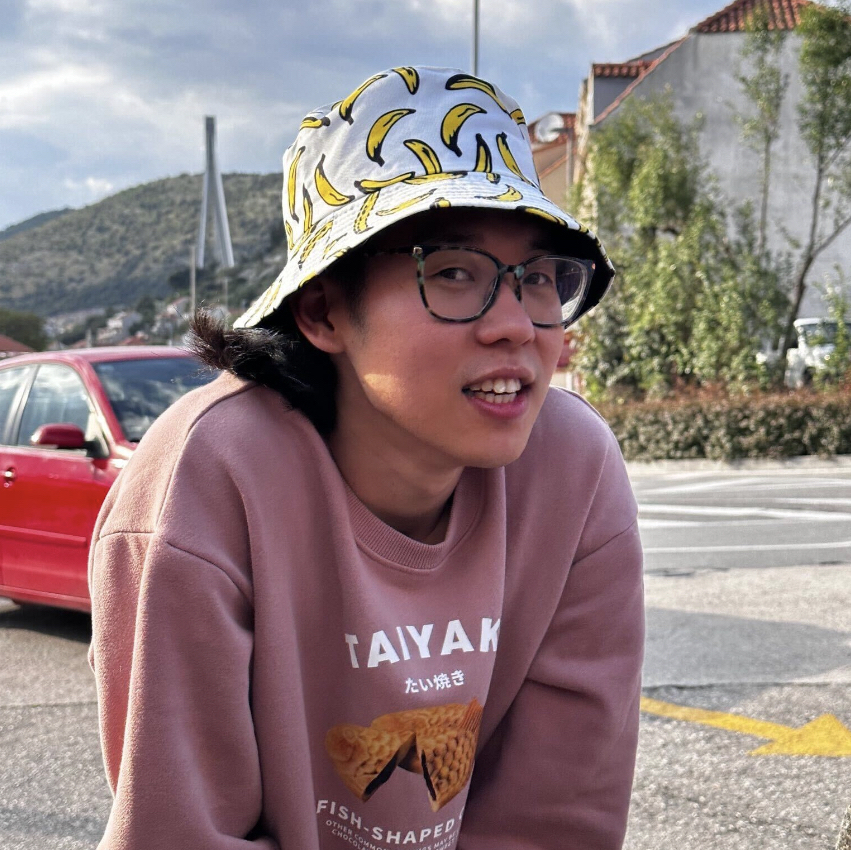
research
I’m a Lead Scientist at the Allen Institute for AI working on the OLMo and Semantic Scholar projects. I specialize in topics in natural language processing, machine learning and human-AI interaction:
me
I live in Seattle. When not working, I hang with my cat Belphegor and play board games (Robinson Crusoe, Cthulu: Death May Die, Hanabi) and video games (Baldur's Gate 3, Valheim, Slay the Spire, Noita, Vampire Survivors). I love D&D and am currently on year three of a campaign in Eberron. I'm a boba enthusiast and my favorites in Seattle are Xing Fu Tang, TP Tea and Sunright Tea Studio.
news
Feb 1, 2024 | Excited to release our first set of artifacts from the OLMo project 🥳 Want models? Download our open-source weights at 1B and a pair of weights at 7B and 7B scale, trained on different hardware, on Huggingface. We also open-source all our training and inference code. Learn more from our paper. Want data? Download all 3T tokens on Huggingface. We also open-source all our dataset construction tools. Learn more from our paper. |
---|---|
Dec 12, 2023 | Happy to be rounding out the year with a Best Paper Award 🏆 for the EMNLP 2023 System Demo track for PaperMage! Also presenting EMNLP 2023 Main Conference and Findings accepted papers on Decontextualizing for Scientific Document Snippets, Tip-of-the-Tongue Retrieval, and Evaluating Multidocument Summarization with Retrieved Documents. Excited to see all my co-authors in Singapore! |
Jun 15, 2023 | Welcome Summer 2023 interns! Excited to be working directly with Orion Weller, Hyunji Lee, Fangyuan Xu and Hang Jiang! |